AI vs Machine: Understanding the Basics
Did you know the global AI market is expected to hit $126 billion by 2025? This shows how crucial it is to know the difference between artificial intelligence and machine learning. In our tech-filled world, understanding AI vs machine is key for both personal and work life.
This guide will help you get to grips with the basics that set artificial intelligence apart from machine learning. By getting these key terms, you’ll better understand how these technologies shape our lives, work, and the economy.
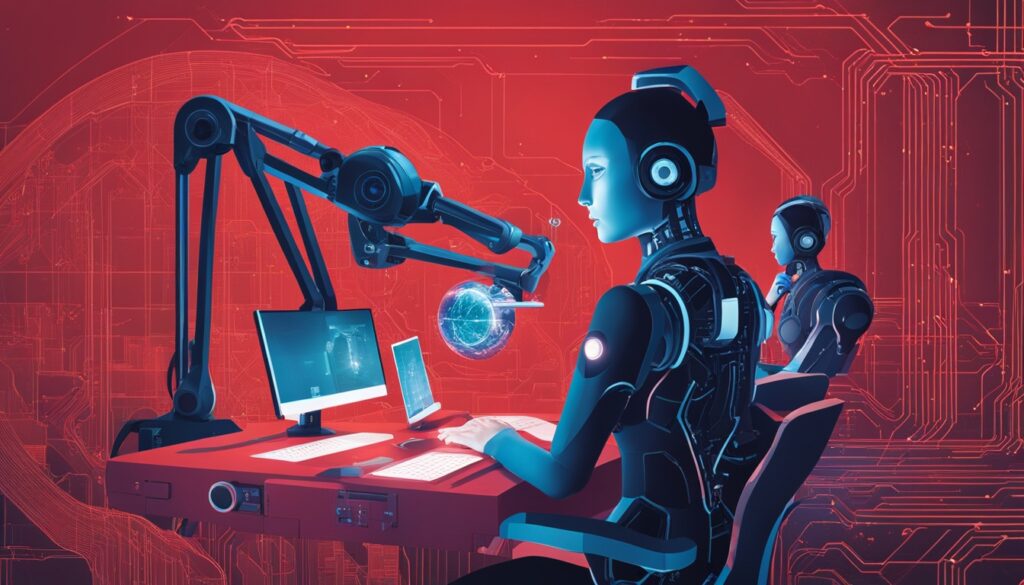
Key Takeaways
- Understanding AI and machine learning is crucial in today’s tech landscape.
- AI refers to systems capable of performing tasks that typically require human intelligence.
- Machine learning is a subset of AI focused on training algorithms to learn from data.
- Comprehending these concepts can help you leverage technology effectively.
- Start exploring the applications of AI and machine learning in various industries.
Introduction to AI and Machine Learning
In the world of tech, learning about AI and machine learning is key. It helps you see how these systems work and what they can do. Artificial intelligence (AI) makes machines think and learn like us. It includes tasks like solving problems, making decisions, and understanding language.
Machine learning is a part of AI. It uses special algorithms to help computers learn from data. This way, machines can get better at tasks like recognizing images or understanding speech over time. It’s crucial for many applications.
Here’s a simple comparison to help you understand the difference:
Aspect | Artificial Intelligence (AI) | Machine Learning (ML) |
---|---|---|
Definition | Simulation of human intelligence in machines | Subset of AI focused on learning from data |
Functionality | Can perform tasks requiring human-like intelligence | Improves performance through experience |
Applications | Wide-ranging, from gaming to autonomous vehicles | Specific tasks like predictions and classifications |
As you learn more about AI basics, it’s crucial to understand these key differences. This knowledge is the base for diving into more complex topics in AI and machine learning.
The Concept of Artificial Intelligence
Artificial intelligence means making computer systems that can do tasks that humans usually do. These tasks include decision-making, *language comprehension*, and visual perception. Real-world examples show how AI works, like self-driving cars, virtual assistants, and chatbots.
What is Artificial Intelligence?
At its core, AI lets machines think like humans do. They solve problems, learn from their mistakes, and adjust to new situations. There are narrow AI for specific tasks and general AI for more complex thinking. Both types show big leaps in tech and the effort to make machines think like us.
History and Evolution of AI
The story of AI started in the 1950s with dreams of machine smarts. Alan Turing’s Turing Test tested if a machine could think like a human. The 1980s brought expert systems, and neural networks made big strides in AI.
Then, deep learning changed everything, letting machines understand huge amounts of data and spot complex patterns. This led to a huge leap in AI, from ideas to real-life uses that change our world. Knowing AI’s history helps us see where it’s going next.
Understanding Machine Learning
Machine learning is a key part of artificial intelligence. It lets systems get better over time by learning from data. To really understand machine learning, we need to look into its definition of ML and the ways it works. This helps us see how tech improves on its own without being told exactly what to do.
What is Machine Learning?
The definition of ML says it’s about making algorithms that let computers learn from data. This means machines find patterns in data and use them to make decisions. This is useful in many areas, like recognizing images or predicting what customers might do.
How well machine learning works depends a lot on the quality and amount of data it uses. Good data is key to making accurate predictions.
Types of Machine Learning
Machine learning has three main types: supervised, unsupervised, and reinforcement learning. Here’s a quick look at each:
Type | Description | Example |
---|---|---|
Supervised Learning | Trains a model on labeled data, where the right answer is known. | Spam detection in email services. |
Unsupervised Learning | Works with data that doesn’t have labels, helping the model find patterns and groups. | Customer segmentation based on what they buy. |
Reinforcement Learning | Teaches models to make decisions by rewarding them for good results. | Game AI that gets better as it plays. |
These types show how machine learning can be used in different ways. Knowing the difference between supervised and unsupervised learning helps us see how each type works in its own way.
AI vs Machine: Understanding the Basics
Artificial intelligence (AI) and machine learning (ML) work together closely, driving tech forward. They are like two sides of the same coin. Knowing how they work together and apart helps us understand the ai vs machine basics.
AI is about making machines think like humans. Machine learning is a part of AI that uses data and algorithms to learn like us. Not every AI uses machine learning, but all machine learning is part of AI.
This shows how each field is unique yet connected. For instance, AI’s natural language processing often gets better with machine learning. Knowing about AI and ML shows how progress in one area helps the other.
Aspect | Artificial Intelligence | Machine Learning |
---|---|---|
Definition | Enables machines to perform tasks that typically require human intelligence. | A subset of AI that focuses on learning from data to enhance performance. |
Scope | Covers various technologies, including reasoning, problem-solving, and natural language processing. | Involves training algorithms to learn patterns and make predictions. |
Data Usage | May use predefined rules without relying on large data sets. | Requires substantial data to train predictive models effectively. |
Examples | Expert systems, chatbots, and computer vision. | Recommendation systems, fraud detection, and autonomous vehicles. |
Looking into these areas helps deepen your knowledge on the ai vs machine basics. It shows where each tech fits in the digital world.
The Differences Between AI and Machine Learning
It’s important to know the difference between AI and machine learning to understand their roles and how they work together. AI is about machines doing tasks that need human smarts. Machine learning is a part of AI that uses algorithms to get better at tasks through experience and data. Here are the main differences to help you see how AI and ML are not the same thing.
Key Distinctions
Aspect | Artificial Intelligence | Machine Learning |
---|---|---|
Definition | Encompasses systems designed to perform tasks requiring human-like intelligence. | A subset of AI that focuses on algorithms to learn from and make predictions based on data. |
Scope | Covers multiple areas, including reasoning, problem-solving, and understanding. | Primarily concerned with pattern recognition and data-driven analysis. |
Examples | Robotics, natural language processing, and expert systems. | Spam detection, recommendation systems, and predictive analytics. |
Common Misconceptions
Many people misunderstand AI and ML, which can lead to confusion. For instance, some think AI can replace human intelligence, but that’s not true. AI and humans work together. Another common mistake is thinking machine learning is the same as AI. Machine learning is just a part of AI, focusing on algorithms to improve through data.
By clearing up these misconceptions, we get a better understanding of AI and ML and their effects on different areas.
Applications of AI in Everyday Life
Artificial intelligence has become a big part of our daily lives. It makes things easier and more efficient. From personal assistants that listen to what you say to new tech in healthcare, AI is changing how we use technology. It also makes our lives better.
AI in Personal Assistants
AI personal assistants like Siri, Google Assistant, and Alexa have changed the game. They use smart language and voice recognition. This lets you do tasks just by talking to them.
You can set reminders, play music, or find information easily. They even suggest things you might like, based on what you do. This shows how AI makes everyday tasks simpler.
AI in Healthcare
AI is making a big difference in healthcare. It helps doctors make better diagnoses and take care of patients. AI uses advanced tech to look at images and predict what patients might need.
Virtual health assistants use learning machines to help patients right away. This makes healthcare better and more accessible. It’s leading to better health for everyone.
Application | Description | Benefits |
---|---|---|
AI Personal Assistants | Voice-activated systems for personal productivity. | Increased efficiency, hands-free operation. |
Predictive Analytics | Data analysis to forecast healthcare trends. | Proactive healthcare management, cost savings. |
AI Imaging Technologies | Tools for improved diagnostic imaging. | Enhanced accuracy, faster diagnostics. |
Virtual Health Assistants | AI agents providing patient support remotely. | Increased accessibility, continuous patient engagement. |
Applications of Machine Learning
Machine learning has changed many industries, like finance and marketing. It shows how ML helps businesses work better.
ML in Financial Services
In finance, machine learning algorithms are key to better decision-making. They help with:
- Credit Scoring: ML makes credit assessments more accurate, reducing lending risks.
- Fraud Detection: Machine learning quickly spots suspicious transactions.
- Algorithmic Trading: ML optimizes trading strategies for better results.
ML in Marketing and Advertising
Machine learning changes marketing by giving deep insights into what customers want. Brands use these insights for better marketing, leading to successful campaigns. Here’s how ML helps in advertising:
- Personalized Experiences: ML offers custom recommendations based on what customers like.
- Targeted Advertising: ML helps find the right audience for ads, boosting engagement.
- Optimized Ad Placements: ML analyzes data to find the best places for ads.
Application Area | Machine Learning Applications | Benefits |
---|---|---|
Financial Services | Credit Scoring, Fraud Detection, Algorithmic Trading | Improved decision-making, enhanced risk assessment |
Marketing and Advertising | Personalized Experiences, Targeted Advertising, Optimized Placements | Increased engagement, tailored customer interactions |
A Comprehensive AI vs ML Comparison
Exploring technology, it’s key to know the differences between artificial intelligence (AI) and machine learning (ML). Understanding these differences helps us see what each can do well and where they fall short.
AI and ML are crucial for today’s innovations. AI is a wide range of tech that tries to act like human intelligence. It includes things like solving problems and learning. Machine learning is a part of AI. It’s about using algorithms to learn from data without needing to be told how.
Here’s a table that highlights the main differences and similarities between AI and machine learning:
Parameter | Artificial Intelligence | Machine Learning |
---|---|---|
Definition | Broader field encompassing various technologies for intelligent behavior | Subset focused on learning from data and improving performance |
Functionality | Simulates human cognitive functions | Analyzes patterns in data to make predictions |
Applications | Can include robotics, natural language processing, and vision systems | Used in recommendation systems, fraud detection, and image recognition |
Technology | Includes expert systems, neural networks, and reasoning engines | Focuses on algorithms such as decision trees and neural networks specific to data |
This detailed look shows that AI and machine learning are closely linked but not the same. They have different ways of working and different uses. Learning about these technologies gives us insight into how they can help us in many areas.
AI and Machine Learning Explained with Examples
Real-world AI examples show how these technologies fit into different industries. They change how we travel and shop, making our lives easier. These examples highlight the tech behind them and how they can improve lives.
Real-World Examples of AI
Many sectors use AI to do better and make things easier for users. Here are some key AI examples:
- Autonomous vehicles use AI to navigate and make quick decisions, making driving safer and more convenient.
- Smart assistants like Siri and Alexa use voice tech to understand what you say, making daily tasks simpler.
- Fraud detection systems in finance use AI to check transactions and spot suspicious ones fast.
Real-World Examples of Machine Learning
Machine learning is big in many areas, changing how things work and interact with users. Here are some examples:
- Netflix’s recommendation system looks at what you watch to suggest shows you might like, making it more fun.
- Amazon’s personalized shopping experience uses ML to suggest products based on what you’ve looked at and bought.
- Email filtering systems use machine learning to sort out the important emails from the spam, saving time.

The Future of AI and Machine Learning
The world of technology is always changing, especially with artificial intelligence (AI) and machine learning (ML). New AI trends are coming up that will change the future of AI and ML. These changes will make industries better and solve hard problems in new ways.
Trends to Watch
Big changes are coming that will change how we use technology. Some of the main trends include:
- Natural Language Processing (NLP): Improvements in NLP will make machines understand human language better. This means better virtual assistants and chatbots.
- Computer Vision: AI is getting better at seeing and understanding pictures. This will help in healthcare, cars, and security.
- Autonomous Systems: Self-driving cars and drones are becoming more common. This means more things will work on their own in different areas.
Challenges Ahead
As AI and ML move forward, we’ll face some big challenges. These challenges include:
- Ethical Implications: Using AI raises questions about who is responsible, if it’s fair, and if it’s biased.
- Data Privacy Concerns: Using more data means more privacy risks. We need strong rules to protect our data.
- Job Displacement Fears: AI might change jobs a lot, making people talk about how to help workers adapt.
Knowing these future hurdles will help us deal with the tough times ahead. It will help us move forward with technology in a smart way.
Trend | Description | Potential Impact |
---|---|---|
Natural Language Processing | Improves machine understanding of human language | Enhances communication in virtual assistants |
Computer Vision | Allows machines to interpret and analyze visual data | Advances applications in safety and health |
Autonomous Systems | Self-operating technologies, such as drones and vehicles | Revolutionizes transportation and delivery services |
The Role of Data in AI and Machine Learning
Data is key in AI and machine learning, driving progress and innovation. Big data is crucial because it lets algorithms learn from lots of information. This leads to better insights in fields like healthcare, finance, and marketing.
Importance of Big Data
Big data is vital for AI and machine learning to work well. It lets systems learn from a wide range of data, spot patterns, and adapt to new situations. This makes them better at making decisions and finding new solutions to tough problems.
Challenges with Data Quality
Even with its benefits, data quality issues in AI and ML are big problems. Bad data, like biased or incomplete sets, can give wrong results. It’s crucial to have clean data to train models on reliable info. You might face problems like:
- Biases in datasets that make results unfair and keep old inequalities.
- Inaccuracies that lead to bad decisions and hurt trust in AI.
- Missing values that make outputs less reliable.
Fixing these data quality issues is key to better performance and results. Good data management helps avoid risks and lets you use big data fully in AI and machine learning.
How to Get Started with AI and Machine Learning
Starting with AI and machine learning can feel daunting because there’s so much to learn. But, with a clear plan, you can make it easier and fun.
Here are some steps to help you get started:
- Online Courses: Websites like Coursera, edX, and Udacity have courses for beginners. Look for ones on Python, statistics, and data science basics.
- Books: “Hands-On Machine Learning with Scikit-Learn, Keras, and TensorFlow” by Aurélien Géron is a great read. It offers practical insights into machine learning.
- Communities: Joining communities like Kaggle or Reddit’s r/MachineLearning can connect you with others. You can share ideas and find mentors.
Practice is crucial as you start learning. Take part in coding challenges and projects. This will help you understand and get more confident in learning machine learning concepts.
Here’s a table to guide you through key topics and resources:
Learning Topic | Suggested Resources | Notes |
---|---|---|
Python Programming | Codecademy, Coursera | Foundation for most machine learning libraries. |
Statistics & Mathematics | Khan Academy, MIT OpenCourseWare | Crucial for understanding algorithms. |
Data Science Fundamentals | DataCamp, edX | Practical applications are vital. |
Machine Learning Algorithms | Udacity, Stanford University’s online course | Delve into supervised and unsupervised learning. |
Real-World Projects | Kaggle Competitions, GitHub | Hands-on experience with real datasets. |
Remember, starting with getting started in AI requires patience and hard work. It takes time, but it can open doors to exciting career paths.
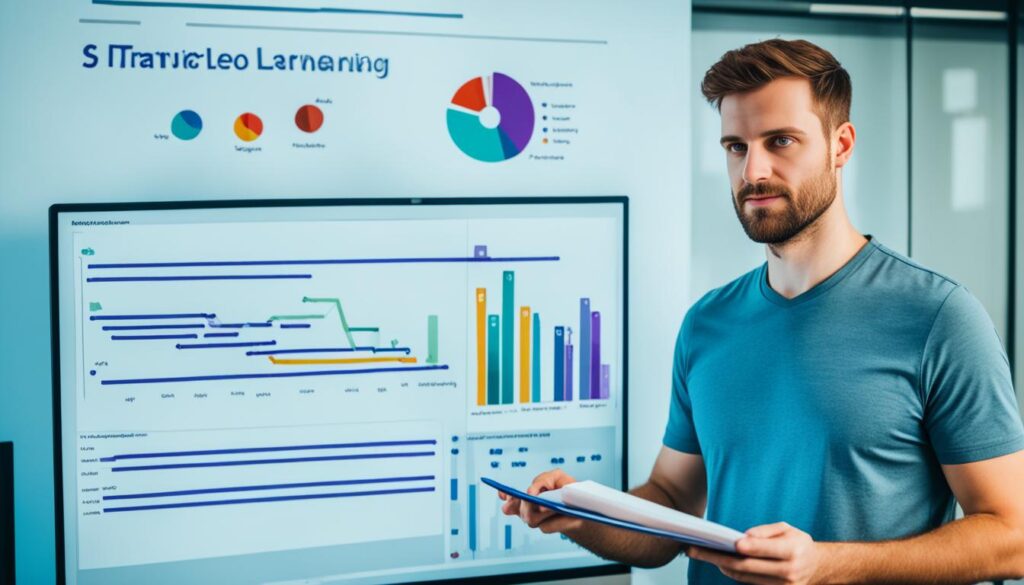
Conclusion
As we wrap up our look at AI and machine learning, let’s think about what we’ve learned. We’ve covered the main points of AI vs ML, showing how they work together. These technologies are key to changing the future of many fields and our daily lives.
Knowing the differences and similarities between AI and machine learning will guide you in a world filled with technology. AI is a powerful tool for making new things and doing things better, with many uses that will make our lives and work better. Machine learning adds to this by making systems that get better with time by learning from data.
FAQ
What is the difference between AI and machine learning?
AI means machines that can do tasks that need human smarts, like solving problems. Machine learning (ML) is a part of AI. It uses stats to help machines learn from data and make predictions.
Can you provide examples of AI and machine learning?
Sure! AI includes virtual assistants like Siri and chatbots. Machine learning is seen in Netflix’s recommendation system. It looks at what you watch to suggest new shows you might like.
What are common misconceptions about AI and machine learning?
Many think AI will replace humans, but it actually helps us. Also, some mix up machine learning with AI. Machine learning is a type of AI, but not all AI uses machine learning.
How does machine learning work?
Machine learning uses algorithms to look at data, find patterns, and make decisions. It gets better over time with more data.
What is the role of data in AI and machine learning?
Data is key in AI and machine learning. Good data is needed for training AI and machine learning models. Bad data can lead to wrong results and bias.
What are the main trends to watch in the future of AI and machine learning?
Look out for new advances in natural language processing and computer vision. Also, ethical frameworks for AI are being developed. These changes will shape our tech future.
How can I get started with AI and machine learning?
Start with online courses, books, and join AI and ML communities. Sites like Coursera, Udacity, and edX offer great resources and certifications to boost your skills.
WORTH READING : https://aibasic.io/top-ai-tools-for-beginners-in-2024-easy-start/
One Comment on “AI vs Machine: Understanding the Basics”